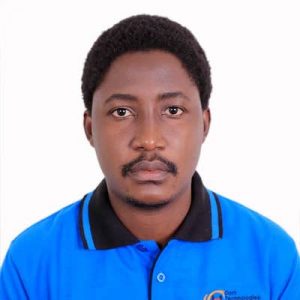
Alfred Ssekagiri
H3Africa PI: David Kateete
Institution: Makerere University
Project Affiliation: BRecA
Abstract
Introduction/Background: Drug resistance poses a significant challenge in the treatment of HIV-1 infection, highlighting the need for the identification of novel drug resistance mutations. Traditional methods for detecting such mutations are often time-consuming and demand substantial expertise. However, machine learning techniques present a promising avenue to tackle this challenge by harnessing the computational power and data-driven algorithms at our disposal.
Objectives: In this study, we aimed to utilize machine learning to identify potentially novel drug resistance mutations in HIV-1 integrase. Additionally, we aimed to interpret the identified mutations and explore their implications on the structural on HIV-1 integrase.
Methodology: We obtained a diverse dataset of 11,962 HIV-1 consensus sequences from the Los Alamos HIV database, including samples labeled as naive or treated. Stanford HIVdb provided drug resistance reports for mutation information. The dataset was encoded using one-hot encoding. To classify the samples into naive and treated categories, we employed three machine learning algorithms: Support Vector Machines (SVM), Random Forest (RF), and Gradient Boosted Machines (GBM). The performance of the machine learning algorithms was evaluated using three metrics which include: sensitivity, specificity, and the area under the receiver operating characteristic curve (AUROC). Additionally, we conducted feature importance analysis to identify top potential novel drug resistance mutations in the integrase gene. The structural implications of these mutations were explored through modelling of mutant structures of integrase using i-TASSER and visualized with PyMOL.
Results/Conclusions: Random Forest (RF) was the best performing algorithm, achieving AUROC of 97%, sensitivity of 99% and specificity of 84%. Feature importance analysis identified top potential novel drug resistance mutations, including G134N, I135V, and K136Q in the integrase gene. Preliminary structural modeling revealed slight conformational changes in the mutant integrase proteins, suggesting potential impacts on drug binding. These findings contribute to our understanding of drug resistance in HIV-1 integrase and provide insights for further research and therapeutic development.
Next steps: Expanding the dataset to include more sequences from sub-Saharan Africa to increase the generalizability of the results.