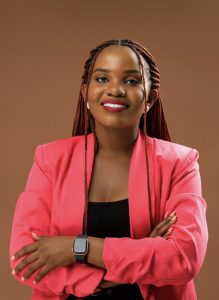
Maria Magdalene Namaganda
H3Africa PI: David Kateete
Institution: Makerere University
Project Affiliation: BRecA
Abstract
HIV/AIDS remains a significant global health issue, with an estimated 38.0 million individuals infected with the virus, despite the major advancements in managing the disease through antiretroviral therapy (ART). Virological failure, one of the challenges in HIV management, often associated with the development of drug-resistance, occurs when ART fails to suppress and sustain a person’s viral load to less than 200 copies/ml. Additionally, HIV is a rapidly evolving virus making it difficult to effectively treat and control. This has led to a growing interest in developing tools and methods for accurately predicting drug-resistance, as early detection and intervention can help to prevent the development of resistance and improve patient outcomes.
A vast amount of data, including genetic information, demographic details and clinical information, is accumulated from regular medical care of HIV patients and is stored in the form of medical records accessible to both healthcare providers and researchers. Ending the HIV epidemic requires innovative use of such data for intelligent decision-making from surveillance through treatment.
Recently, Machine learning (ML) has emerged as a promising approach for predicting virological failure in HIV. ML has the ability to identify and discover patterns in complex datasets including genetic, epidemiological, lifestyle, behavioral, and clinical data and offers a principled approach for developing algorithms for the analysis of high-dimensional and multimodal biomedical data.
We aim to optimize HIV patient-care through integrated analysis of routinely collected data with a purpose of predicting virological failure using machine learning in a resource-limited setting, and to explore the potential of this approach to improve our understanding of HIV treatment outcomes and inform clinical decision-making.
We expect to develop and validate an accurate, efficient, and easy-to-use machine learning-based model to predict virological failure among HIV patients; and demonstrate the potential of this approach to improve our understanding of drug resistance and inform clinical decision-making, particularly in resource-limited settings.
Keywords: HIV/AIDS, virological failure, HIV drug resistance, machine learning, predictive models, resource-limited settings